ROBUST-6G
UMU CyberDataLab Decentralised Federated Learning for joint privacy-preserving AI/ML model training
The main objective of this use case is to design a comprehensive Decentralized Federated Learning (DFL) framework to facilitate AI trustworthiness assessment within highly distributed network topologies. This framework aims to guide the creation of AI models through federated schemes that avoid central entities and servers, in contrast to current centralised solutions that are not optimised for advanced distributed 6G environments. For AI/ML model generation, the cloud, fog, edge and extreme edge nodes in each network share AI/ML model updates with nodes in the other networks, training the shared models using a DFL approach. In addition, this use case aims to evaluate the trustworthiness of AI/ML models developed using the DFL above approach by examining basic principles such as accountability, fairness, explainability and robustness, along with critical aspects of federated learning such as privacy. Different scenarios and verticals can, therefore, be evaluated in this use case.
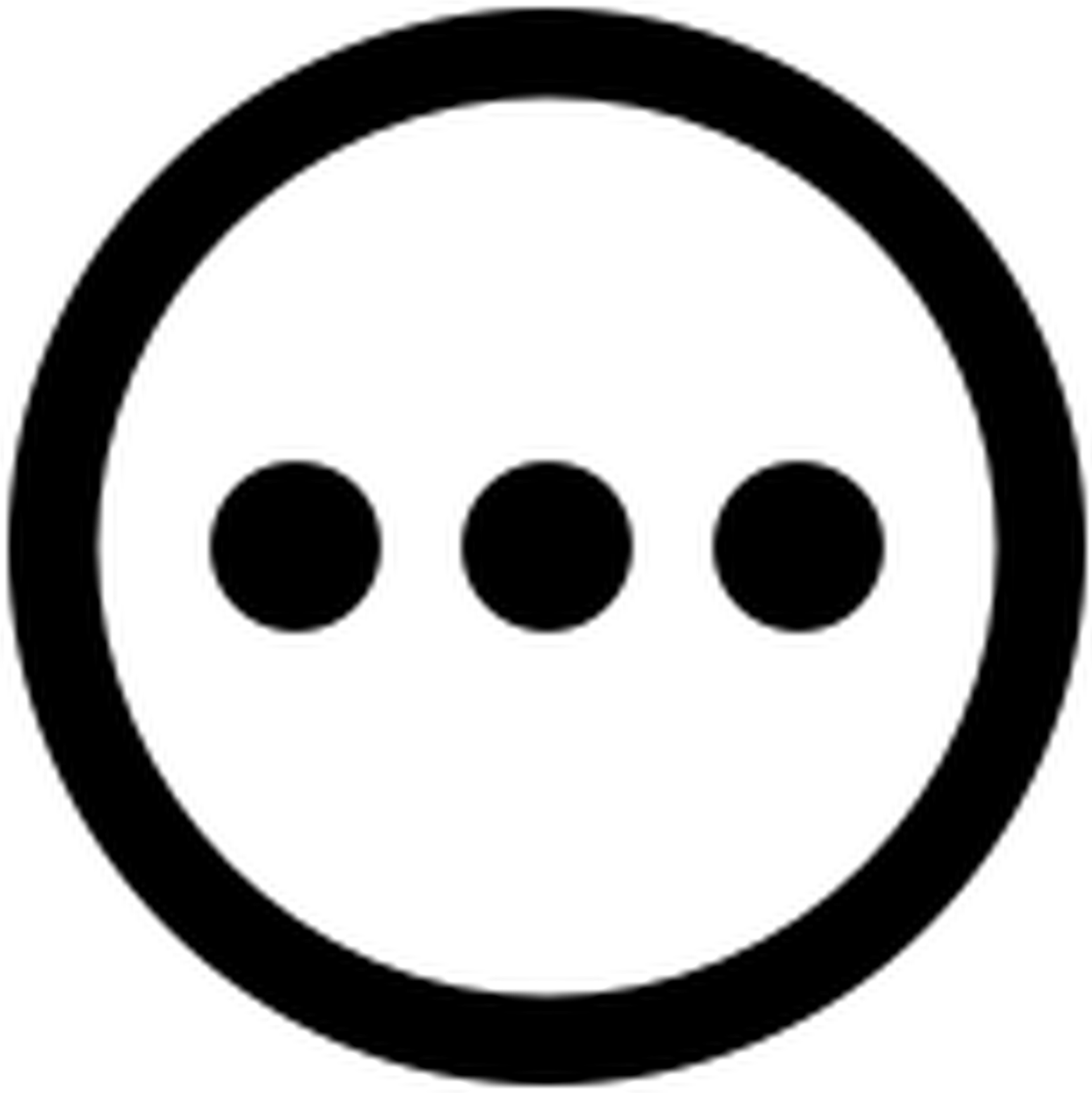
ROBUST-6G
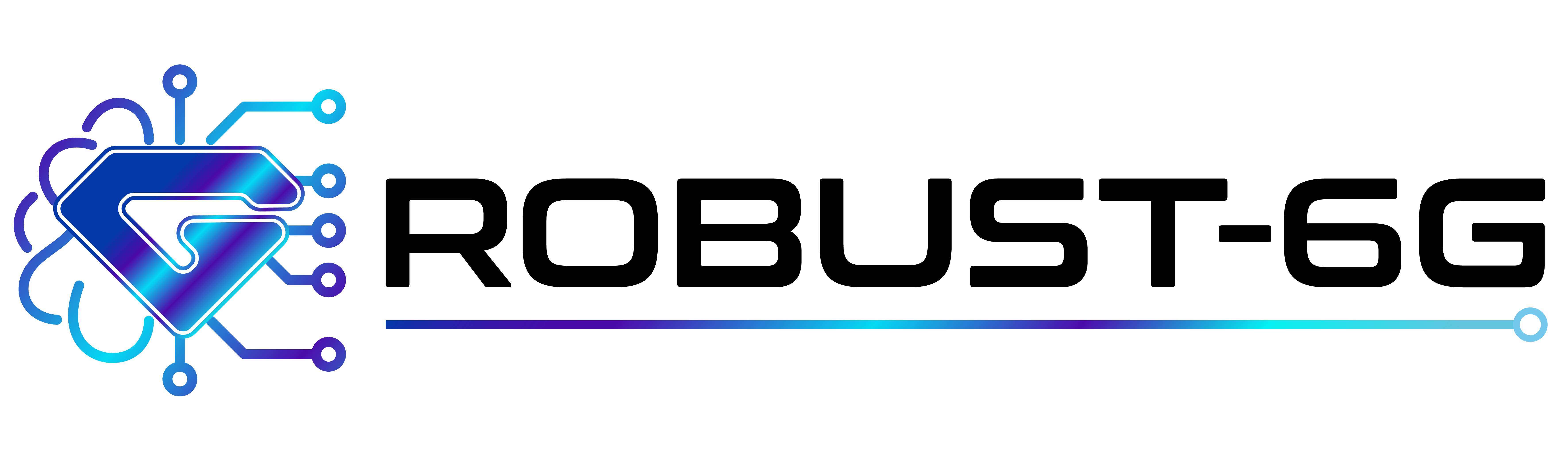