ORIGAMI
Distributed User-Plane Inference
Deploying Machine Learning (ML) models in the user plane enables low-latency and scalable in-network inference, but integrating them into programmable devices faces stringent constraints in terms of memory resources and computing capabilities. In this demo, we showcase a solution for user-plane ML developed within the ORIGAMI project and named DUNE. DUNE is a novel framework for distributed user-plane inference across multiple programmable network devices through the automated decomposition of large ML models into smaller sub-models. DUNE mitigates the limitations of traditional monolithic ML designs that fit ML models into single network devices. We run experiments on a testbed with five Intel Tofino programmable switches using measurement data and show how DUNE not only improves the accuracy that the traditional single-device monolithic approach gets but also maintains a comparable per-switch sub-millisecond latency.
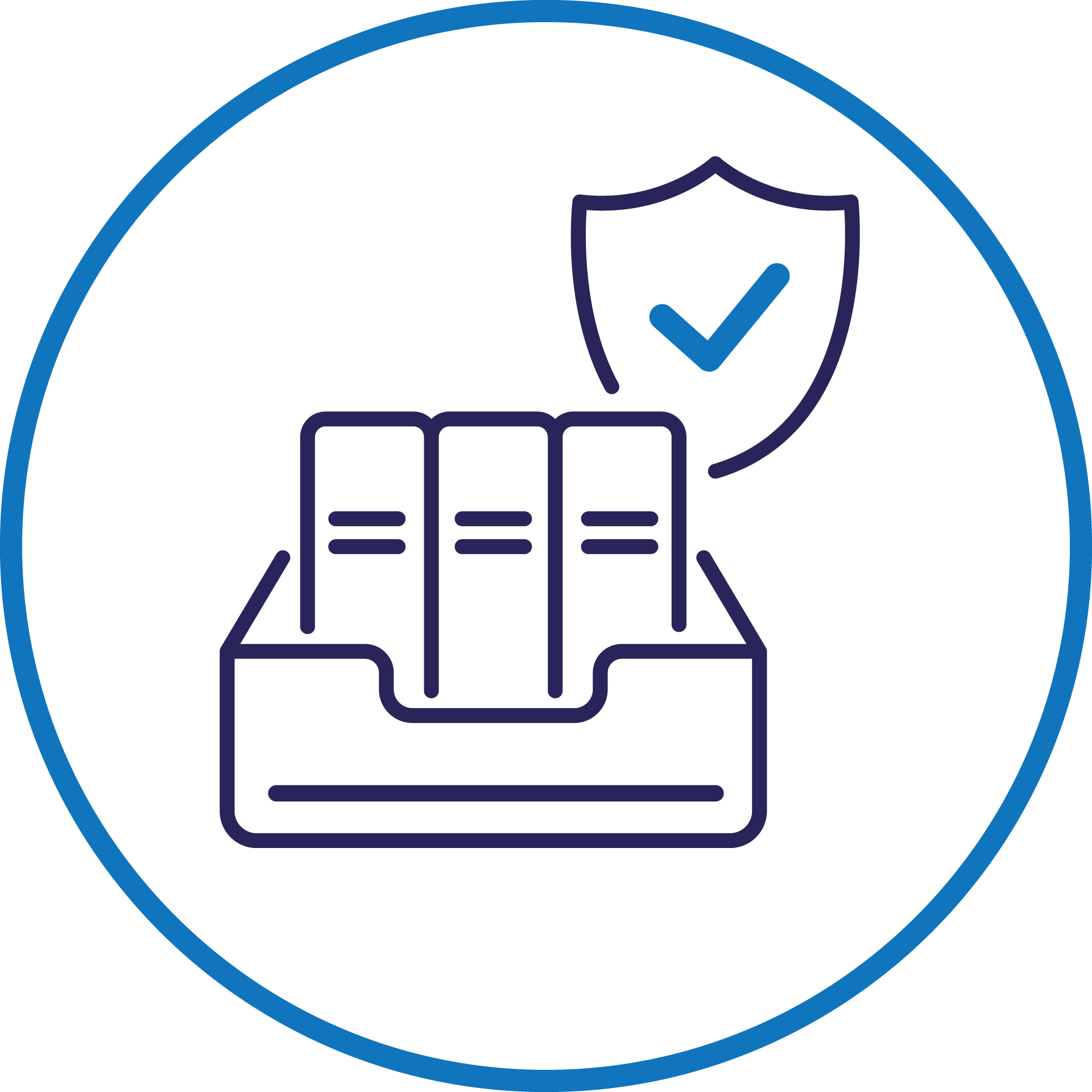
ORIGAMI
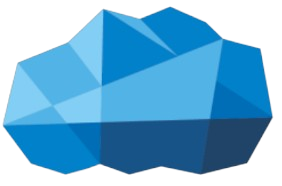