6G-TWIN
Energy savings in dense deployments supported by network digital twins
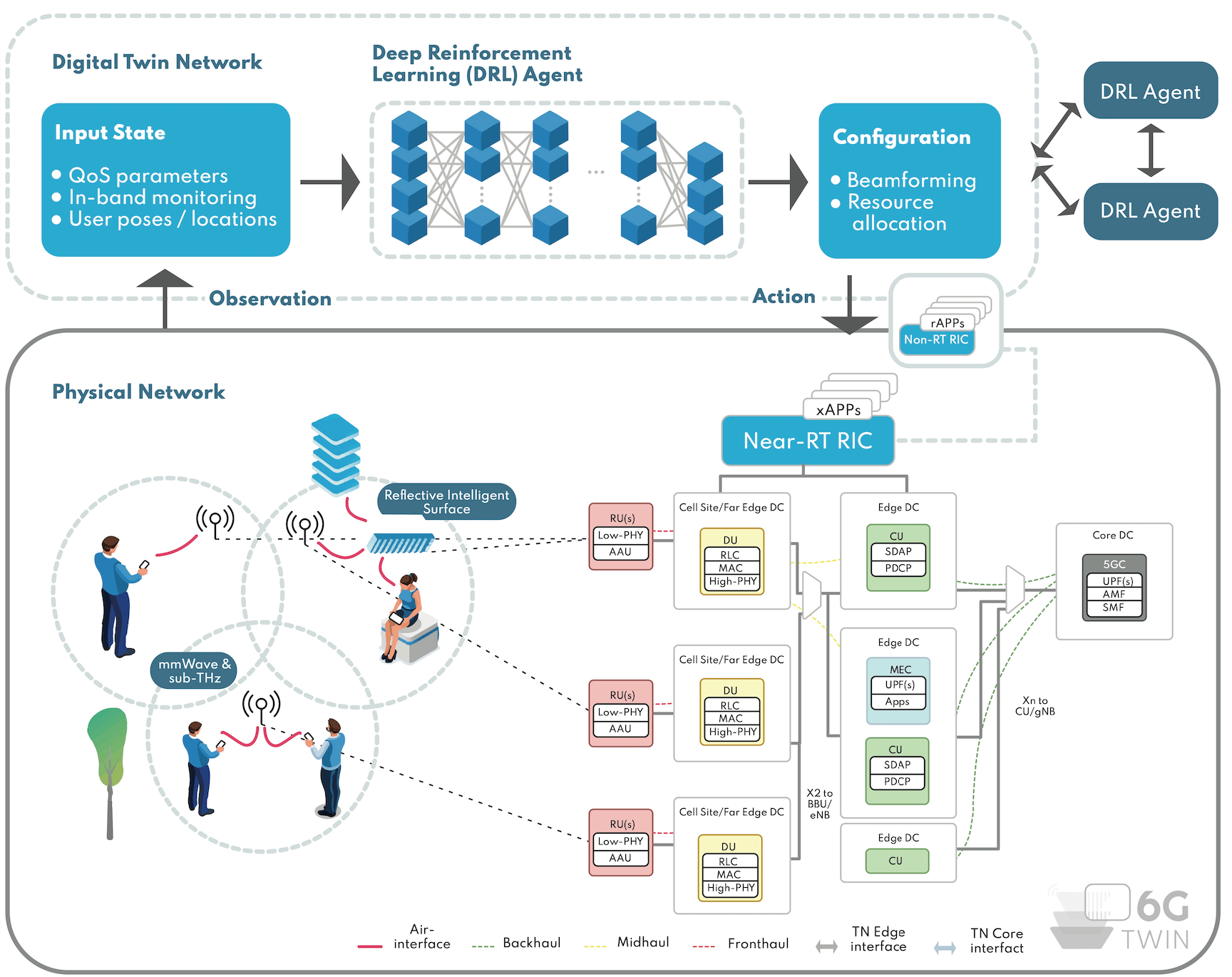
This use-case targets a green approach to mobile networks, which provides increased efficiency and better energy usage by optimising network resources. Data-driven networks, mainly empowered by AI/ML, or hybrid approaches between ML, analytical models and mathematical models, will be required to optimise performance in such complex scenarios and supported by open architectures to avoid vendor locks, enhance flexibility and increase innovation and adoption.
In this use-case, 6G-TWIN’s Network Digital Twin (NDT) is fed by data collected across the network (RAN, Edge, Core). Specifically, it uses QoS parameters defined for the end users, system performance data provided by in-band monitoring, and localisation/sensing data (e.g., user poses and locations). The NDT generates a range of RAN scenarios replicating real-life traffic patterns and generates the ORAN O2 data set to train the DRL agent. The DRL agent triggers x-/r-apps related to changing power consumption at different levels to save energy in densely populated areas. The near-RT xApps implemented in near-RT RIC are responsible for Cell and Beam level optimisations on short-term (milli-second), while rApps are responsible for non-RT long-term actions, such as nightly power-down management.
Three complementary scenarios of increasing complexity will be explored to evaluate energy efficiency.
In this use-case, 6G-TWIN’s Network Digital Twin (NDT) is fed by data collected across the network (RAN, Edge, Core). Specifically, it uses QoS parameters defined for the end users, system performance data provided by in-band monitoring, and localisation/sensing data (e.g., user poses and locations). The NDT generates a range of RAN scenarios replicating real-life traffic patterns and generates the ORAN O2 data set to train the DRL agent. The DRL agent triggers x-/r-apps related to changing power consumption at different levels to save energy in densely populated areas. The near-RT xApps implemented in near-RT RIC are responsible for Cell and Beam level optimisations on short-term (milli-second), while rApps are responsible for non-RT long-term actions, such as nightly power-down management.
Three complementary scenarios of increasing complexity will be explored to evaluate energy efficiency.
Type of experiment:
Simulation/Emulation
Functionality:
Intelligent Network Architecture
Location(s):
Belgium
Vertical sector(s):
Smart Energy
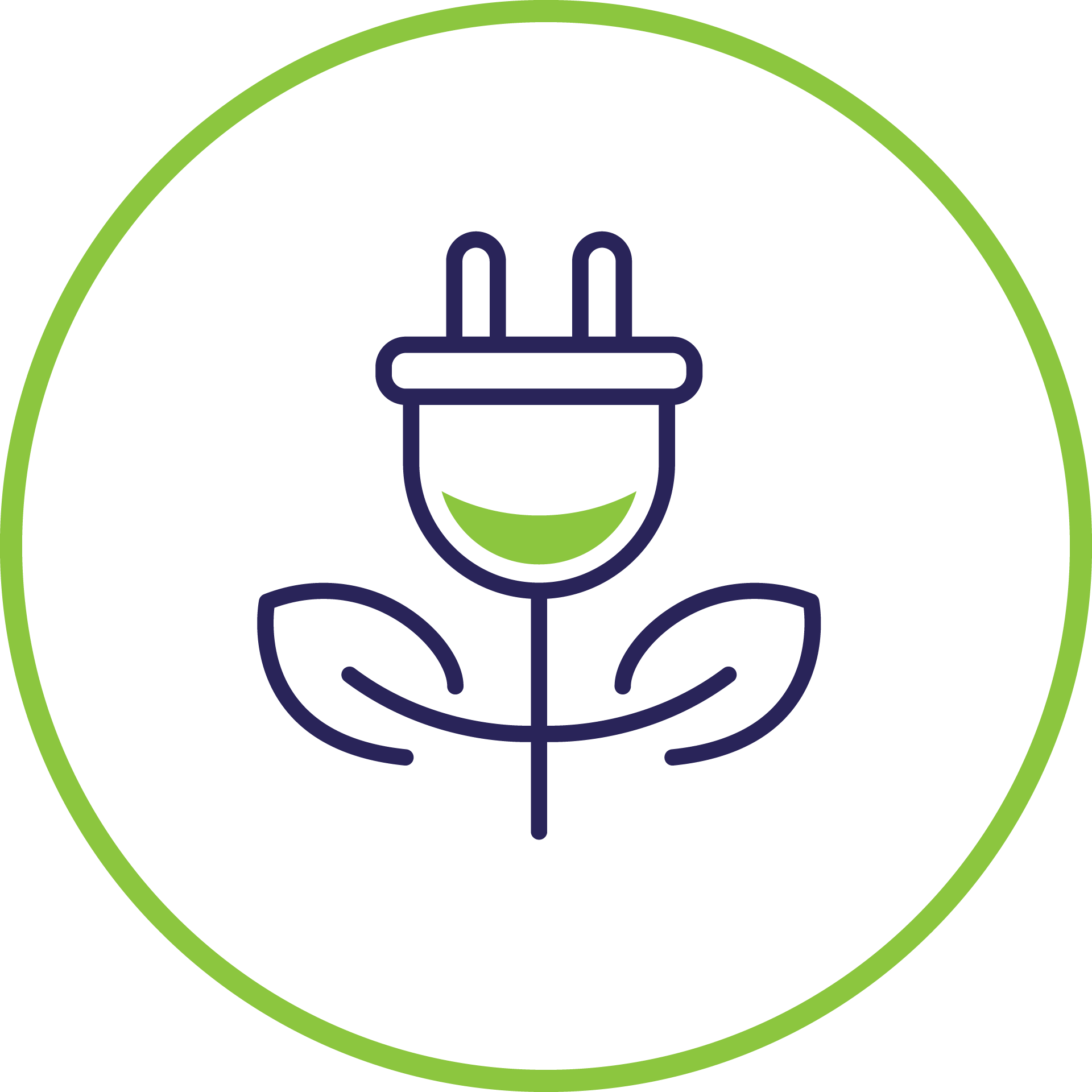
6G-TWIN
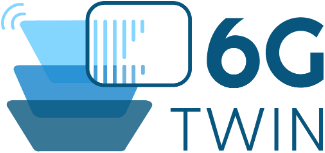
Duration:
GA Number:
101136314
SNS JU Call (Stream):
Call 2